1. What is Agriculture Technology (AgTech)?
In the field of agricultural technology (AgTech), a wide range of technologies and innovations is developed to enhance the effectiveness, productivity, and sustainability of agricultural practices. These technologies include tools like precision farming equipment, automated irrigation systems, drones, and artificial intelligence (AI) systems. One essential task in agriculture is fruit sorting, where fruits are categorized based on their colour, size, and health condition. Fruit sorting is critical for farmers, fruit traders, and agricultural researchers as it helps with harvest planning, managing the supply chain, and predicting market prices. Traditionally, fruit sorting methods have been labour-intensive and prone to inaccuracies, which can vary from person to person. However, with the advent of AI, computer vision, and deep learning, there is an opportunity to significantly automate and improve this process. In this blog, we explore how state-of-the-art AI models can be used for precise fruit sorting based on colour, size, and health condition.
2. What is AgTech for Fruit Sorting?
AgTech for fruit sorting encompasses the utilization of autonomous or semi-autonomous robots, drones, and AI-based software for automation. AgTech systems consist of a combination of different hardware components such as robots and drones, alongside various AI-based models. A robotic arm equipped with an AI system can be used to detect, and analyze the fruit instances placed on the moving chain. Usch Agricultural robots, also known as agribots or agri-robots, hold the potential to revolutionize the farming industry by undertaking a wide spectrum of tasks. Some of the diverse functions that agricultural AI systems, robots, and drones can perform include:
2.1. Quality Grading:
AI systems can assess fruit quality by examining factors like colour consistency, texture, and overall appearance. This helps in categorizing fruits into different quality grades for market purposes.
2.2. Size Classification:
AI algorithms can measure the size of fruits accurately, ensuring they meet specific size requirements for packaging and sale.
2.3. Color Sorting:
By analyzing the colour of fruits, AI can separate them based on ripeness or colour uniformity. This aids in organizing fruits for different market segments or processing purposes.
2.4. Texture Assessment:
AI can evaluate the texture of fruits, distinguishing between firm and soft fruits, which is crucial for packaging and distribution.
2.5. Health Condition Monitoring:
AI systems can identify signs of disease or damage on fruits, enabling early detection and removal of unhealthy fruits to prevent the spread of diseases.
2.6. Packaging Optimization:
AI helps optimize the packaging process by ensuring that fruits of similar size, quality, or ripeness are grouped together for packaging, reducing packaging costs.
2.7. Harvest Planning:
AI can predict the optimal time for fruit harvesting based on factors like colour change and size growth, helping farmers plan their harvest efficiently.
2.8. Pest and Disease Detection:
Drones equipped with AI can survey orchards for signs of pest infestations or disease outbreaks, allowing for targeted intervention.
2.9. Yield Estimation:
AI models can estimate fruit yields by analyzing data from sorting and monitoring, aiding in production planning and resource allocation.
2.10. Post-Harvest Handling:
Robots can be employed for gentle handling of fruits after harvest, reducing bruising and damage during transportation.
2.11. Market Segmentation:
Fruits can be sorted according to consumer preferences, allowing for customized packaging and marketing strategies for different market segments.
2.12. Data Analytics:
AI systems collect valuable data during the sorting process, which can be used for trend analysis, crop improvement, and decision-making in subsequent growing seasons.
These functions not only improve the efficiency of fruit sorting but also contribute to reduced waste, improved product quality, and enhanced overall productivity in the agricultural industry.
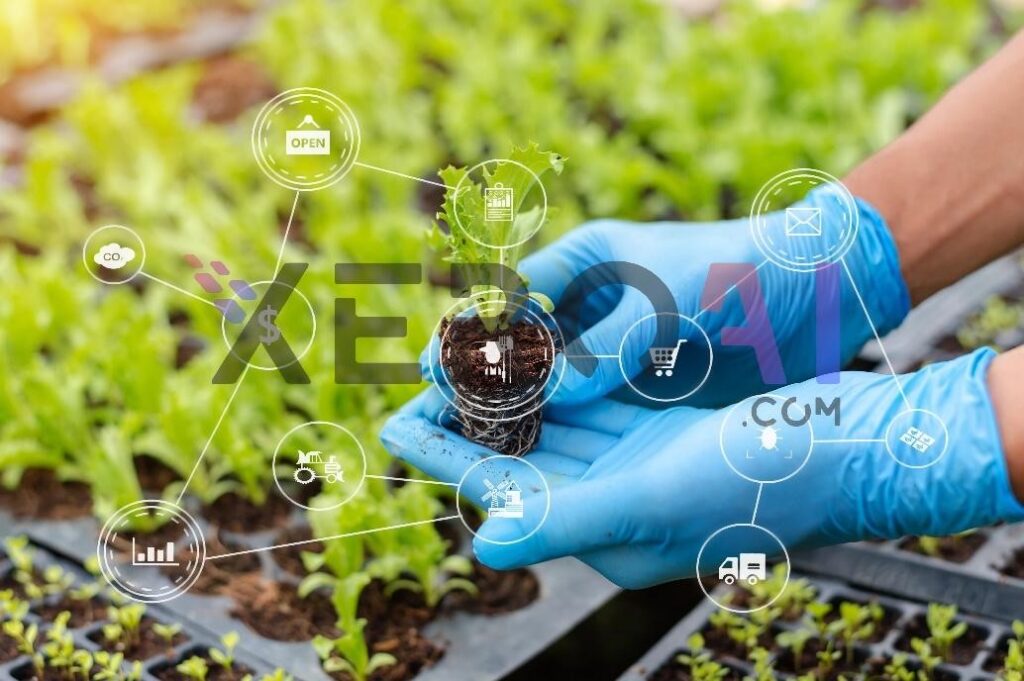
Figure 1: Utilization of AgTech in Different Applications
3. Benefits of AgTech in Fruit Sorting
AgTech is a cornerstone of modern farming methods, offering a valuable tool for elevating the effectiveness and precision of fruit sorting based on colour, size, and health condition. This technological advancement not only enhances decision-making processes but also contributes to greater sustainability in agriculture. As technology continues to advance, AgTech is poised to play an increasingly pivotal role in revolutionizing various facets of the agricultural sector. The sorting of fruits in orchards holds substantial significance in agriculture for the following compelling reasons: Storing fruits based on colour, texture, size, and health condition offers several benefits in terms of improved fruit quality, reduced waste, and better marketability. Here are some of the key benefits:
3.1. Enhanced Fruit Quality:
Sorting fruits based on these criteria ensures that only fruits meeting specific quality standards are stored. This results in a higher overall quality of stored fruits, making them more appealing to consumers.
3.2. Extended Shelf Life:
By segregating fruits based on their ripeness and health condition, it becomes possible to store fruits optimally. This can lead to an extension of their shelf life, reducing spoilage and waste.
3.3. Reduced Waste:
Effective sorting helps identify fruits with defects, diseases, or overripeness. By separating these fruits, the risk of contamination and spoilage within storage is minimized, reducing overall waste.
3.4. Customized Packaging:
Sorting allows for customized packaging based on size, color, or quality. This can cater to different market segments, improving marketability and potentially leading to higher prices.
3.5. Improved Handling:
Uniformly sized and textured fruits are easier to handle during packaging and transportation. This reduces the risk of physical damage, bruising, and loss during handling.
3.6. Optimized Storage Conditions:
Fruits with similar characteristics can be stored together, ensuring that they are subjected to the most suitable storage conditions, such as temperature and humidity levels.
3.7. Efficient Inventory Management:
With fruits sorted by various criteria, it becomes easier to track inventory and manage stock levels. This can prevent overstocking or understocking of specific fruit types.
3.8. Marketability:
Well-sorted fruits are more visually appealing and meet consumer preferences, leading to increased sales and marketability.
3.9. Minimized Disease Spread:
Separating diseased or damaged fruits from healthy ones can help prevent the spread of diseases and infections within storage facilities.
3.10. Data-Driven Decisions:
Sorting systems often collect data on fruit quality and characteristics. This data can be analyzed to make informed decisions about future planting, harvesting, and storage strategies.
3.11. Resource Efficiency:
By optimizing the storage of fruits, resources such as storage space and cooling facilities can be used more efficiently, potentially reducing operational costs.
3.12. Consumer Satisfaction:
Ultimately, well-sorted fruits lead to greater consumer satisfaction, as they receive high-quality, fresh produce that meets their expectations.
Sorting fruits based on color, texture, size, and health condition not only improves fruit quality and shelf life but also has economic and environmental benefits by reducing waste and optimizing resource usage. It contributes to a more efficient and sustainable fruit storage and distribution system.
4. AgTech in fruit sorting and monitoring system
AgTech in fruit sorting and monitoring refers to the application of advanced technologies including AI, machine learning (ML), computer vision (CV), robotics, and other digital tools to automate, optimize, and improve the methods of sorting and monitoring fruits. These methods are designed to address various challenges faced in the traditional farming methods of fruit sorting and monitoring. Here’s a brief look at how AgTech plays a role in these areas:
4.1. Fruit Sorting
Traditional fruit sorting methods often rely on human labour and are time-consuming, costly, and error-prone. AgTech, on the other hand, uses sophisticated AI and computer vision models that proces the camera stream to automate this process. The fruits are sorted based on several parameters such as size, colour, shape, health condition and potential defects. High-resolution camera and sensors are used to capture images of different fruits, which are then processed and analyzed by AI models. The models have been trained to identify and categorize different fruit types and conditions, making the sorting process faster, more accurate, and more efficient. Then robots and robotics arms are used as hardware tool to perform that sorting peractically. In short, AI model does the intelligent work to identify the desired fruit instances and robotics arms perform the action.
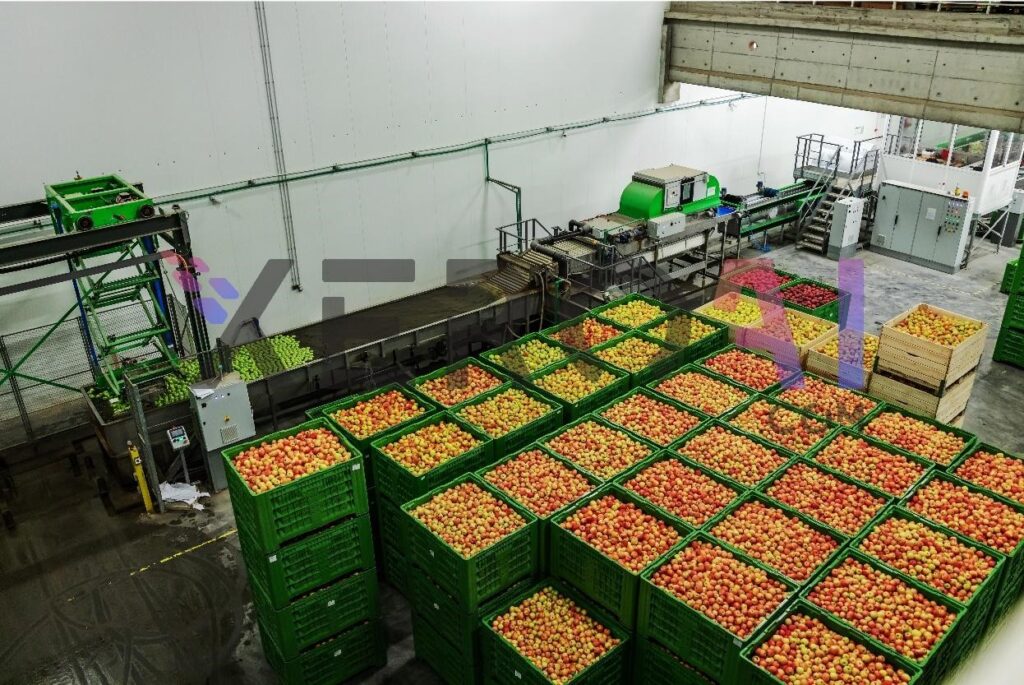
Figure 2: Traditional Fruit Sorting Method
4.2. Fruit Monitoring
Monitoring fruit growth and health status is a critical part of fruit farming. Using AgTech, this process can be significantly enhanced. For instance, drones or satellites equipped with high-resolution sensors can capture images of an entire orchard. These images can then be analyzed using deep learning models to monitor the fruits’ health and identify signs of diseases, pest infestations, or drought stress. The use of technology in the process of fruit monitoring allows for real-time monitoring and early detection of potential challenges, enabling farmers to take timely corrective actions. This leads to healthier crops, improved yields, and reduced losses. Even with the robotic arm, AI models can helpout to identity such fruit instances that were overlooked by the human labour.
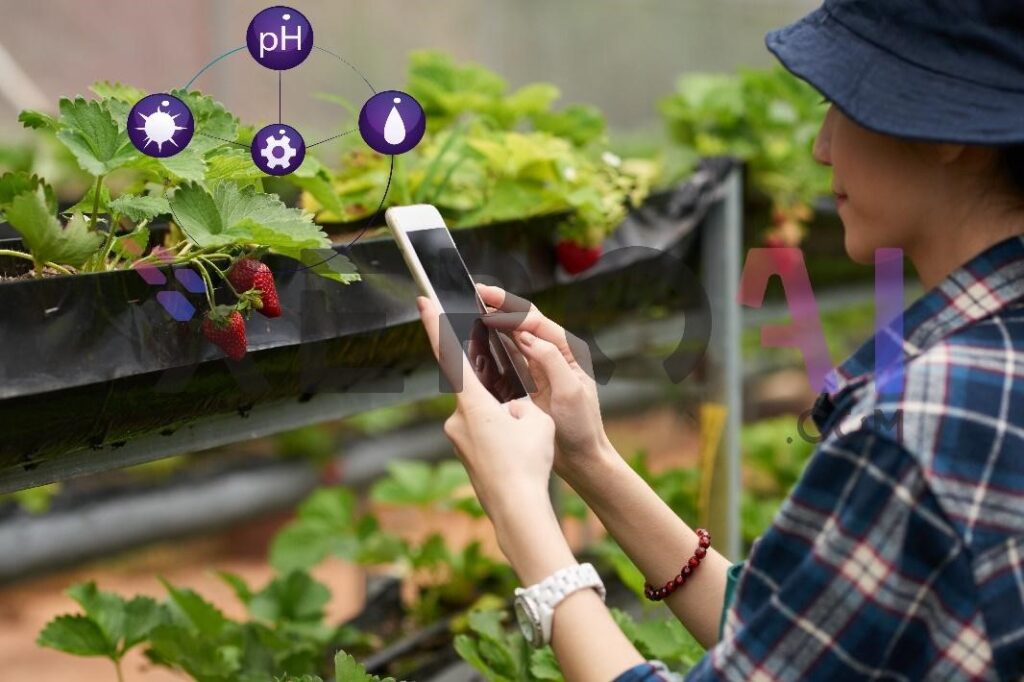
Figure 3: Fruit Monitoring Method with AgTech
5. Process of AI System for Fruit Sorting and Monitoring
This process is composed of AI software module and robotic arms. AI model is the mind of the system, while robotics module is the body to perform the action:
5.1. Data Collection
The first step in training EfficientNet for fruit sorting and monitoring is data collection. High-quality images of various fruits in different states are needed. This may include images of fruits of different types, sizes, colours, stages of ripeness, and different kinds of defects or diseases. High-resolution cameras are typically used to capture the fruit images. For fruit monitoring, images can be captured using drones or satellites. This allows for the collection of images that show various perspectives, from close-ups of individual fruits to aerial views of an entire orchard. In this blog, we will use images of different fruits taken from an open-source repository.
5.2. Data Augmentation
Data augmentation involves creating additional training samples by applying various transformations to the original images. This might include rotations, flips, zooms, and shifts. This technique helps to increase the amount of training data and also to reduce overfitting by providing a more diverse set of samples for the model to learn from.
5.3. Data Annotation
After data collection data annotation (data labeling) has been performed. This step involves tagging each image in the dataset with the appropriate labels such as size, colour, and health condition. For fruit monitoring, the images would be labelled based on factors such as the health status of the plants, signs of disease, or pest infestation. There are several tools available for data annotation, ranging from open-source tools like LabelImg to more sophisticated ones that support large-scale, collaborative annotation efforts.
5.4. Deep Learning Model Selection
Selecting the optimal deep learning model for fruit sorting based on size, color, texture, and health condition requires a balance between accuracy and inference time efficiency. Several Convolutional Neural Network (CNN) architectures have proven effective for computer vision tasks, and the choice depends on your specific requirements and constraints. Here, we’ll explore some popular CNN models and discuss which one might be the best fit for your fruit sorting application:
5.4.1. ResNet (Residual Networks):
- Accuracy: ResNet is known for its exceptional accuracy, even in very deep networks. It has won several image recognition competitions.
- Inference Time Efficiency: While ResNet models are accurate, they are relatively deeper, which can make them slower for inference compared to some shallower architectures. However, optimizations like model quantization can help mitigate this.
5.4.2. MobileNet:
- Accuracy: MobileNet is designed for efficiency and is considered lightweight compared to models like ResNet. While it may not achieve the same accuracy as ResNet, it can still provide good results for many tasks.
- Inference Time Efficiency: MobileNet is specifically optimized for mobile and edge devices. It’s much faster in terms of inference time compared to deeper architectures like ResNet. It’s a great choice if you need real-time or near-real-time processing.
5.4.3. EfficientNet:
- Accuracy: EfficientNet represents a family of models with a balance of accuracy and efficiency. Depending on your resource constraints, you can choose from different model sizes (e.g., B0 to B7) to achieve the desired trade-off between accuracy and efficiency.
- Inference Time Efficiency: EfficientNet models are efficient and can provide a good balance between accuracy and inference time efficiency. The smaller versions are faster and can still perform well on many tasks.
5.4.4. DenseNet (Densely Connected Convolutional Networks):
- Accuracy: DenseNet is known for its strong performance in accuracy. It has dense connections between layers, allowing for better feature reuse and learning.
- Inference Time Efficiency: DenseNet models tend to be deeper and, as a result, might be slower in inference compared to some lighter architectures like MobileNet.
5.4.5. SqueezeNet:
- Accuracy: SqueezeNet is designed to be compact yet accurate. It achieves good results while having a significantly smaller model size compared to deeper networks.
- Inference Time Efficiency: SqueezeNet is highly efficient in terms of inference time, making it a good choice for resource-constrained environments.
5.4.6. ShuffleNet:
- Accuracy: ShuffleNet balances accuracy and efficiency by using channel shuffle operations to reduce computational complexity.
- Inference Time Efficiency: ShuffleNet is designed for efficient inference and can be a suitable choice for real-time applications.
It’s important to note that model performance also depends on the size and quality of your dataset, as well as the specifics of your hardware and deployment environment. It’s often a good practice to experiment with a few different models, fine-tune them on your data, and evaluate their performance based on your criteria for accuracy and inference time efficiency before making a final selection.
6. Implementation Challenges and Solutions
The primary challenge is the need for a large, high-quality, labeled dataset for training the model. Additionally, mutli-labels prediction requires substantial model changes because trained AI model will predict one class, while our desired system need to perform prediction against fruit size, color, texture, health condition and defects in fruit instaces. Moreover, Attributes like color, texture, and defects can interact with each other. For example, a change in color may be indicative of a defect. Your mowever, these challenges can be addressed. For the data requirement, data augmentation techniques can be used to artificially increase the size of the dataset.
7. Conclusion
The AI model holds great promise for agriculture, especially in areas like fruit sorting and monitoring. It offers a high level of accuracy while maintaining efficiency, surpassing traditional manual or semi-automatic methods. While the initial investment in data collection, model training, and infrastructure setup may be substantial, the long-term benefits of increased productivity, better decision-making, improved fruit quality, and labor cost reduction make it a worthwhile endeavor. With continued advancements in AI models will continue to revolutionize agriculture, providing solutions that were previously unimaginable. The future of agriculture is indeed high-tech and promising.